Note
Go to the end to download the full example code.
Training a SFNO on the spherical Shallow Water equations
In this example, we demonstrate how to use the small Spherical Shallow Water Equations example we ship with the package to train a Spherical Fourier-Neural Operator
import torch
import matplotlib.pyplot as plt
import sys
from neuralop.models import SFNO
from neuralop import Trainer
from neuralop.training import AdamW
from neuralop.data.datasets import load_spherical_swe
from neuralop.utils import count_model_params
from neuralop import LpLoss, H1Loss
device = torch.device('cuda:0' if torch.cuda.is_available() else 'cpu')
Loading the Spherical Shallow Water Equations in multiple resolutions
train_loader, test_loaders = load_spherical_swe(n_train=200, batch_size=4, train_resolution=(32, 64),
test_resolutions=[(32, 64), (64, 128)], n_tests=[50, 50], test_batch_sizes=[10, 10],)
Loading train dataloader at resolution (32, 64) with 200 samples and batch-size=4
Loading test dataloader at resolution (32, 64) with 50 samples and batch-size=10
Loading test dataloader at resolution (64, 128) with 50 samples and batch-size=10
We create a spherical FNO model
model = SFNO(n_modes=(32, 32),
in_channels=3,
out_channels=3,
hidden_channels=32,
projection_channel_ratio=2,
factorization='dense')
model = model.to(device)
n_params = count_model_params(model)
print(f'\nOur model has {n_params} parameters.')
sys.stdout.flush()
self.sht_grids=['equiangular', 'equiangular']
self.sht_grids=['equiangular', 'equiangular']
self.sht_grids=['equiangular', 'equiangular']
self.sht_grids=['equiangular', 'equiangular']
Our model has 275555 parameters.
Create the optimizer
optimizer = AdamW(model.parameters(),
lr=8e-4,
weight_decay=0.0)
scheduler = torch.optim.lr_scheduler.CosineAnnealingLR(optimizer, T_max=30)
Creating the losses
l2loss = LpLoss(d=2, p=2, reduce_dims=(0,1))
# h1loss = H1Loss(d=2, reduce_dims=(0,1))
train_loss = l2loss
eval_losses={'l2': l2loss} #'h1': h1loss,
print('\n### MODEL ###\n', model)
print('\n### OPTIMIZER ###\n', optimizer)
print('\n### SCHEDULER ###\n', scheduler)
print('\n### LOSSES ###')
print(f'\n * Train: {train_loss}')
print(f'\n * Test: {eval_losses}')
sys.stdout.flush()
### MODEL ###
SFNO(
(positional_embedding): GridEmbeddingND()
(fno_blocks): FNOBlocks(
(convs): ModuleList(
(0-3): 4 x SphericalConv(
(weight): ComplexDenseTensor(shape=torch.Size([32, 32, 32]), rank=None)
(sht_handle): SHT(
(_SHT_cache): ModuleDict()
(_iSHT_cache): ModuleDict()
)
)
)
(fno_skips): ModuleList(
(0-3): 4 x Flattened1dConv(
(conv): Conv1d(32, 32, kernel_size=(1,), stride=(1,), bias=False)
)
)
(channel_mlp): ModuleList(
(0-3): 4 x ChannelMLP(
(fcs): ModuleList(
(0): Conv1d(32, 16, kernel_size=(1,), stride=(1,))
(1): Conv1d(16, 32, kernel_size=(1,), stride=(1,))
)
)
)
(channel_mlp_skips): ModuleList(
(0-3): 4 x SoftGating()
)
)
(lifting): ChannelMLP(
(fcs): ModuleList(
(0): Conv1d(5, 64, kernel_size=(1,), stride=(1,))
(1): Conv1d(64, 32, kernel_size=(1,), stride=(1,))
)
)
(projection): ChannelMLP(
(fcs): ModuleList(
(0): Conv1d(32, 64, kernel_size=(1,), stride=(1,))
(1): Conv1d(64, 3, kernel_size=(1,), stride=(1,))
)
)
)
### OPTIMIZER ###
AdamW (
Parameter Group 0
betas: (0.9, 0.999)
correct_bias: True
eps: 1e-06
initial_lr: 0.0008
lr: 0.0008
weight_decay: 0.0
)
### SCHEDULER ###
<torch.optim.lr_scheduler.CosineAnnealingLR object at 0x7f55f7fc1580>
### LOSSES ###
* Train: <neuralop.losses.data_losses.LpLoss object at 0x7f55f7fc1940>
* Test: {'l2': <neuralop.losses.data_losses.LpLoss object at 0x7f55f7fc1940>}
Create the trainer
trainer = Trainer(model=model, n_epochs=20,
device=device,
wandb_log=False,
eval_interval=3,
use_distributed=False,
verbose=True)
Train the model on the spherical SWE dataset
trainer.train(train_loader=train_loader,
test_loaders=test_loaders,
optimizer=optimizer,
scheduler=scheduler,
regularizer=False,
training_loss=train_loss,
eval_losses=eval_losses)
Training on 200 samples
Testing on [50, 50] samples on resolutions [(32, 64), (64, 128)].
Raw outputs of shape torch.Size([4, 3, 32, 64])
[0] time=3.56, avg_loss=2.6527, train_err=10.6106
Eval: (32, 64)_l2=2.0615, (64, 128)_l2=2.3643
[3] time=3.53, avg_loss=0.4048, train_err=1.6192
Eval: (32, 64)_l2=0.5565, (64, 128)_l2=2.4791
[6] time=3.49, avg_loss=0.2600, train_err=1.0398
Eval: (32, 64)_l2=0.4029, (64, 128)_l2=2.3919
[9] time=3.51, avg_loss=0.2179, train_err=0.8714
Eval: (32, 64)_l2=0.3482, (64, 128)_l2=2.3720
[12] time=3.50, avg_loss=0.1841, train_err=0.7366
Eval: (32, 64)_l2=0.3252, (64, 128)_l2=2.3557
[15] time=3.49, avg_loss=0.1637, train_err=0.6548
Eval: (32, 64)_l2=0.2852, (64, 128)_l2=2.3634
[18] time=3.51, avg_loss=0.1415, train_err=0.5661
Eval: (32, 64)_l2=0.2680, (64, 128)_l2=2.3406
{'train_err': 0.544685577750206, 'avg_loss': 0.1361713944375515, 'avg_lasso_loss': None, 'epoch_train_time': 3.503250279000042}
Plot the prediction, and compare with the ground-truth Note that we trained on a very small resolution for a very small number of epochs In practice, we would train at larger resolution, on many more samples.
However, for practicity, we created a minimal example that i) fits in just a few Mb of memory ii) can be trained quickly on CPU
In practice we would train a Neural Operator on one or multiple GPUs
fig = plt.figure(figsize=(7, 7))
for index, resolution in enumerate([(32, 64), (64, 128)]):
test_samples = test_loaders[resolution].dataset
data = test_samples[0]
# Input x
x = data['x']
# Ground-truth
y = data['y'][0, ...].numpy()
# Model prediction
x_in = x.unsqueeze(0).to(device)
out = model(x_in).squeeze()[0, ...].detach().cpu().numpy()
x = x[0, ...].detach().numpy()
ax = fig.add_subplot(2, 3, index*3 + 1)
ax.imshow(x)
ax.set_title(f'Input x {resolution}')
plt.xticks([], [])
plt.yticks([], [])
ax = fig.add_subplot(2, 3, index*3 + 2)
ax.imshow(y)
ax.set_title('Ground-truth y')
plt.xticks([], [])
plt.yticks([], [])
ax = fig.add_subplot(2, 3, index*3 + 3)
ax.imshow(out)
ax.set_title('Model prediction')
plt.xticks([], [])
plt.yticks([], [])
fig.suptitle('Inputs, ground-truth output and prediction.', y=0.98)
plt.tight_layout()
fig.show()
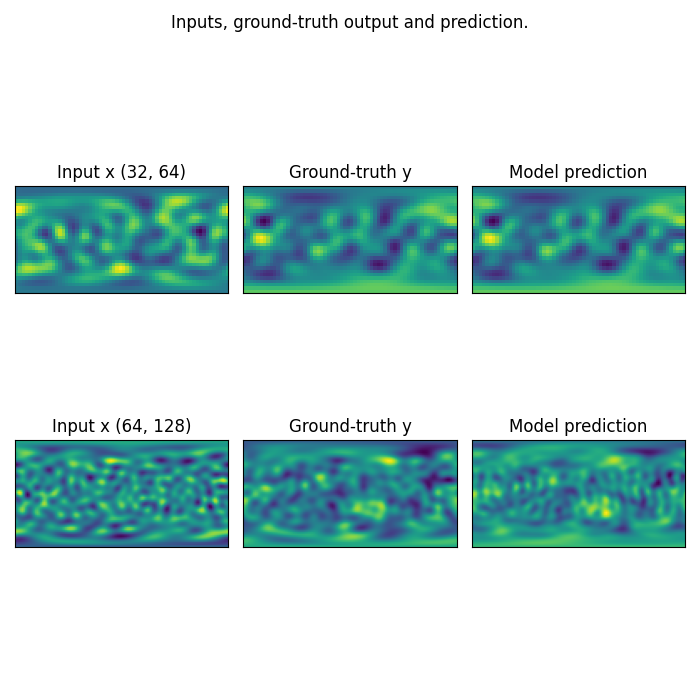
Total running time of the script: (1 minutes 26.183 seconds)